Blog
The Ultimate Guide to the Data Journey from Data to Insights
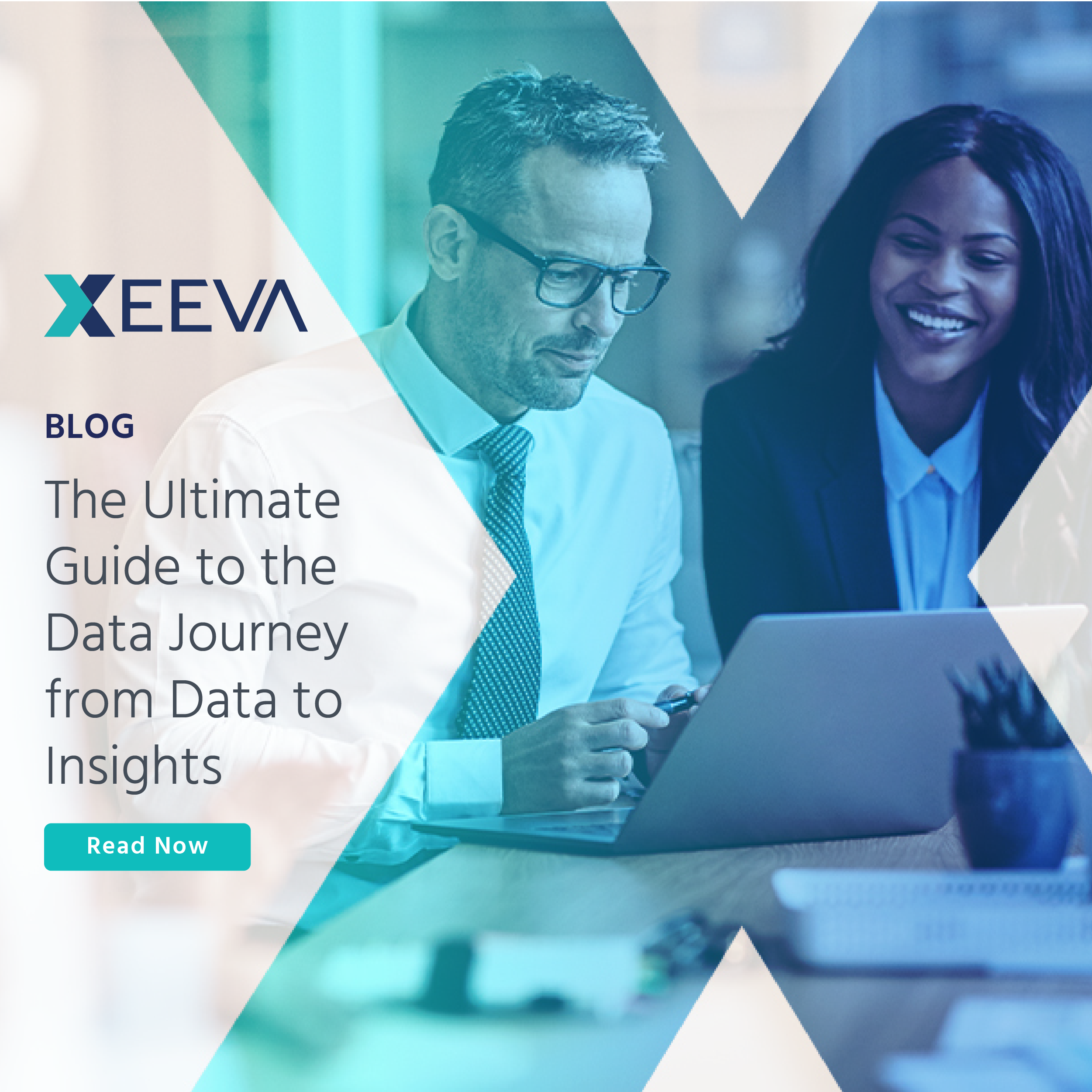
Every human, business, government entity, public or private organization depends on data-based facts and evidence to make day-to-day decisions. Whether quick, agile, based on extended discussions, or based on an in-depth analysis of data, all decisions require supporting data. The question is, where and how does an entity get its underlying data from? And how does an organization process that data to generate information that forms the basis for its decision-making? This is the foundation for the use of data, data analysis, and data-based analytics.
How it has evolved:
Though Big data and Data analytics has become a game-changer for all businesses and organizations across the globe since O’Reilly Media in 2005 coined the term Big Data; its origin and usage can be traced back well before that. The use of data in Statistics has been called upon for thousands of years, as far back as when rulers and kings wanted information about their land, agriculture, commerce, populations of their states to assess their wealth, taxation system, military potential, and various other aspects of government. Data Analytics or Data science came to the forefront when the established science of statistics was coupled with computer science to make an entirely new discipline, which is now termed as the sexiest job of the 21st century.
How to use Data:
Understanding the outcomes of any data-driven technology in alignment with business goals is the key to using the data effectively. The knowledge hidden inside the raw data is surfaced only when the data is given a proper shape, structure, and alignment. The strategies that are used to transform raw data to provide insights must include:
Deciding the Goal:
The collection of data must align with the business goals and objectives. Once the user knows what outcomes are desired from the analysis, the appropriate data can be collected.
Choosing the Data:
The first step is to determine which data to collect from the many available data sources. Since customer data is the core of any business, it is usually a good starting point in most instances. The decision-maker must determine “What customer data is relevant for a particular analysis?”
Collecting the Data:
Once the desired analysis and the relevant data sources are determined, data collection becomes easy. The biggest challenge in data collection is to collect complete data without any missing elements, and errors. It is equally critical to record and saves the complete data without any errors for future use.
Cleansing the data:
Cleansing includes the process of identifying and correcting incomplete and inaccurate data; replacing, modifying, and deleting irrelevant, repetitive, or missing data. In other words, Garbage data should be corrected or removed from the process. This manual cleansing process or formula-driven under the traditional statistical methods is now faster and more accurate by using emergent technologies such as artificial intelligence, machine learning, and algorithms.
Classifying the data:
Classification of data is the start, arguably the first step in data analysis. This involves organizing data into various buckets, clusters, and subcategories based on variables and questions it needs to answer. The classification of data can have various processes or approaches like user-driven classification, automated classification, paper-based classification. Sometimes classification can be based on sensitivity and risk associated with the data; and can be classified as public, internal-only, confidential, and restricted.
Enriching the data:
This process involves adding value to the data. During the processing of data, analysts often find that the data is missing some key elements that assist with classification. The process of identifying, locating, and adding those missing pieces to add overall value is called enrichment. This is often achieved by merging the third-party data with the customer data to make the data more valuable to address the analysis at hand and to surface better insights including visualizations to stakeholders.
Analyzing the data:
Various BI analytical tools and techniques such as Tableau, SAP, ZOHO Analytics, MicroStrategy, programming scripts such as Python and R, and excel spreadsheets play a role in data analysis. The goal of data analytics is to uncover the trends in data. The ability to slice and dice the data using easy-to-use tools and dashboards to help decision-makers analyze the data according to their business need is pivotal. Complex data is made simple via data analytics and BI visualization tools. Adding value to data in addition to data analysis to surface compelling insights can be achieved by:
- Easy-to-understand charts and dashboards make it easier to comprehend complex data. The more the data can be drilled down to the lowest details, the better the appeal and desirability. This can serve as a USP for any analytical tool.
- Flexible data analysis rules support dynamic business conditions. With the business environment being highly dynamic, there are huge shifts in data. Any analysis that reflects the changing data trends is always better than the analysis that only shows a trend fixed in time.
- Establishing relationships and comparisons is another driver for data analysis. Therefore, adding scope for ongoing data refresh and continuous comparisons yields better outcomes.
- Sharing analysis with a larger audience can bring about added brainstorming and different aspects that surfaced from the data analysis. This leads to more holistic and strategic decision-making.
- It is important to bear in mind that data can also mislead if analyzed incorrectly or with incorrect, and incomplete information. Thus, it is important to continuously refresh data, continuously refine data analysis and keep the scope open for further analysis using different aspects.
The more specific the business goals and data, the more accurate the data processing with faster analytical outcomes, and finally, better decision making.
How Xeeva provides Insights driven by customer’s Spend Data
- Xeeva as a company believes in “Better Data, Better Insights, Better Outcomes”. Xeeva is a trusted partner and a vendor of choice for many Industry leaders. It supports its customer from the beginning of their spend journey till the end. From the collection of the spend data onwards, it adds value to the data throughout its journey as it evolves into insights and outcomes. Xeeva’s data-driven AI-powered end-to-end indirect spend management solution provides valuable insights to assist with customer procurement and purchasing decision-making.
- Xeeva classifies spend data into relevant categories using its industry agnostic and proprietary taxonomy, analyzing spend data more meaningful and insightful.
- With Xeeva’s Data Enrichment, aspects of data are enriched to support better sourcing, purchasing, and analytics – making data more valuable and powerful.
- Once classified and enriched, Xeeva analyzes the data using multiple criteria including supplier, category, time horizon, and many other aspects.
- Xeeva succeeds where others fail. Once data is processed, Xeeva’s solution helps customers identify inaccurate and incomplete records, as well as lack of consistency in data and internal procurement processes.
- Xeeva’s sourcing and procurement specialists complement customer’s internal procurement teams in managing and implementing procurement and purchasing best practices. This is Xeeva’s continuous value addition throughout the customer’s journey.
- Insights from data enable a path for bigger and continued savings for customers.
To learn more about Xeeva’s spend management solutions, start your free trial here.